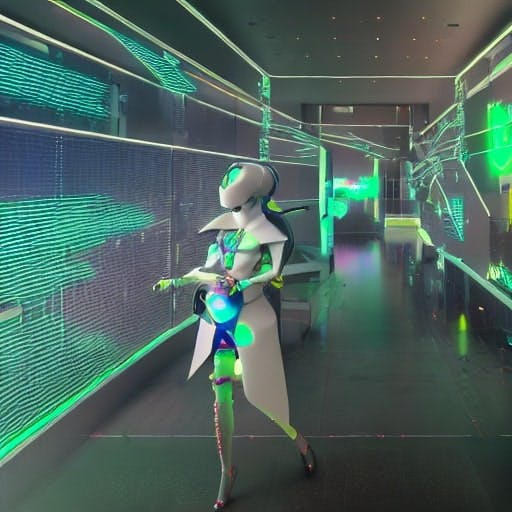
Loading...
Loading...
In order to evaluate the accuracy of our machine learning model on data that it has not come across yet.
In most cases we are just given one data set and its our job to determine how to break that up for training data and what should be used for testing data. That is where Cross validation comes in. Cross validation is a technique used to determine which data set is used for training and which data set is used for testing in an unbiased way.
The data set we feed in to our ML algorithms is divided into two subsets, one for training and one for testing to see how each subset performs against one another.
Basically we then go through all the possible variations of our data in the subsets / folds to determine which data set should be used for training and will response new data with a more generalized prediction.
Training data is what we actully pass in to our ML model to learn and indentify patterns.
Testing data is what we use to indentify the accuracy of our ML model and algorithms.